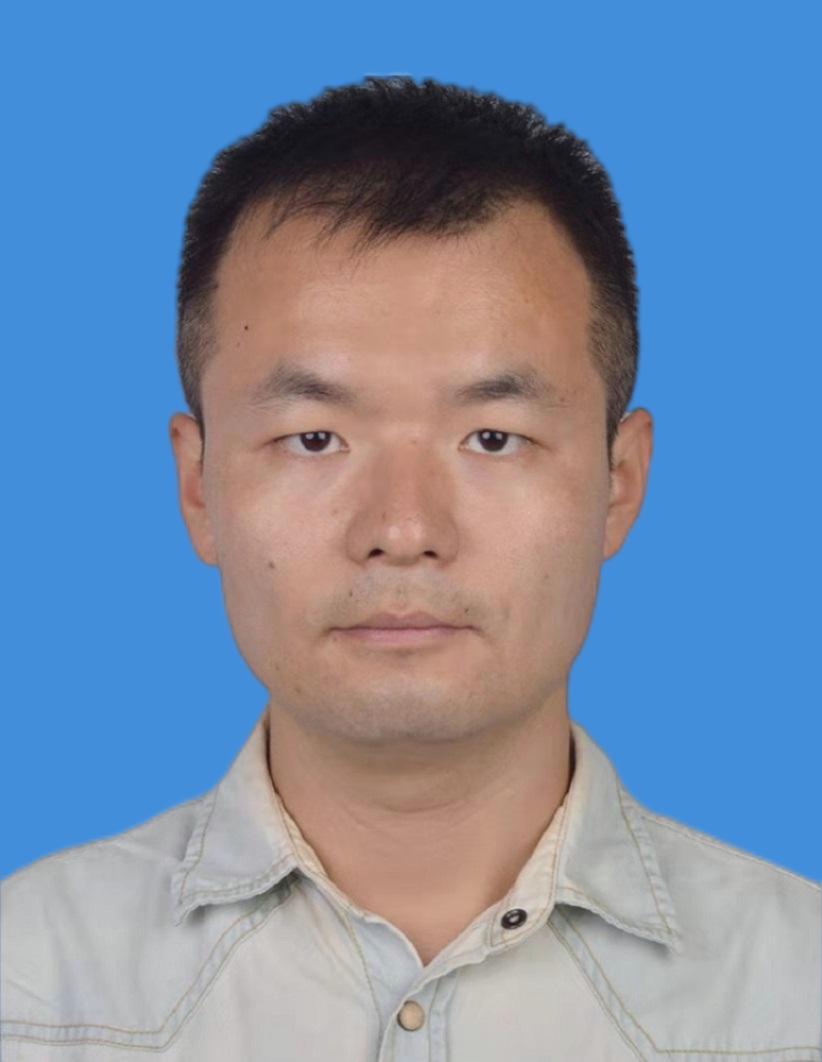
Hi, I'm a postdoctoral researcher at RIKEN AIP in Japan. I got my Ph.D. in the School of Computer Science and Engineering at Nanjing University of Science and Technology (NJUST), where I was lucky to have Prof. Zhong Jin as my supervisor. Prior to joining RIKEN, I held a postdoctoral position at the School of Automation at Guangdong University of Technology (GDUT) under the guidance of Prof. Guoxu Zhou.
My research focuses on advancing machine learning through the exploration of tensor-based theories and models. I specialize in leveraging low-rank tensors to uncover intricate data or parameter patterns, thereby enhancing algorithm performance. My work spans various domains, including trustworthy multi-modal learning and quantum machine learning, where I strive to develop innovative approaches that push the boundaries of current methodologies.
Can tensor learning be as effective as deep learning?
ChatGPT 3.5: It depends on the specific task, the nature of the data, and the available resources. In some scenarios, tensor learning methods may outperform deep learning, especially when dealing with multi-dimensional data with inherent structures that can be effectively captured by tensor models. Additionally, tensor methods may offer advantages in terms of interpretability, computational efficiency, and data efficiency in certain contexts. Therefore, rather than dismissing tensor learning outright, it's essential to consider it as a complementary approach to deep learning, suitable for specific tasks and data characteristics.
Research Experience
2012.9 ~ 2015.6 Autonomous DrivingI possess over two years of research experience in environmental sensing for unmanned ground vehicles. My work, focused on designing and implementing negative obstacle detection algorithms within on-board embedded systems, has significantly honed my practical programming skills.
2015.1 ~ Tensor-based Machine LearningTensor Networks (TNs), with deep roots in quantum physics, chemistry, and applied mathematics, have demonstrated exceptional performance in handling high-dimensional data, generating multiway structured data, optimizing neural network structures and etc. Recently, it is further developed as a potential driving force in advances of machine learning (ML), particularly in crucial areas such as quantum ML, trustworthy ML, and interpretable ML. I am interested in not only the fundamental challenges of TNs such as TN structure optimization, efficient algorithms, and robustness, but also their extended application in addressing key challenges within ML, spanning domains such as efficiency, interpretability, reliability and etc.
Skill, Knowledge, and Capability (with score)
Matrix and Tensor Analysis 70%Selected Publications
[13] Wang, A., Li, C., Bai, M., Jin, Z., Zhou, G., & Zhao, Q. (2023). Transformed low-rank parameterization can help robust generalization for tensor neural networks. Advances in Neural Information Processing Systems (NeurIPS), 2023. [Pdf
link]
[12] Wang, A., Zhou, G., Jin, Z., & Zhao, Q. (2023). Noisy tensor completion via orientation invariant tubal nuclear norm. Pacific Journal Of Optimization, 19(2), 273-313. [Pdf
link]
[11] Luo, Y., Wang, A., Zhou, G., & Zhao, Q. (2022). A hybrid norm for guaranteed tensor recovery. Frontiers in Physics, 10, 885402. [Pdf
link]
[10] Wang, A., Zhao, Q., Jin, Z., Li, C., & Zhou, G. (2022). Robust tensor decomposition via orientation invariant tubal nuclear norms. Science China Technological Sciences, 65(6), 1300-1317. [Pdf
link]
[9] Wang, A., Zhou, G., & Zhao, Q. (2021). Guaranteed robust tensor completion via L-SVD with applications to remote sensing data. Remote Sensing, 13(18), 3671.[Pdf
link]
[8] Wang, A., Zhou, G., Jin, Z., & Zhao, Q. (2021). Tensor recovery via L-spectral k-support norm. IEEE Journal of Selected Topics in Signal Processing, 15(3), 522-534.
[7] Wang, A., Zhou, G., Jin, Z., & Zhao, Q. (2020). An L1-L2 variant of tubal nuclear norm for guaranteed tensor recovery. IJCAI workshops on Tensor Network Representations in Machine Learning, 2020. [Pdf
link]
[6] Wang, A. D., Jin, Z., & Yang, J. Y. (2020). A faster tensor robust PCA via tensor factorization. International Journal of Machine Learning and Cybernetics, 11, 2771-2791.
[5] Wang, A., Li, C., Jin, Z., & Zhao, Q. (2020). Robust Tensor Decomposition via Orientation Invariant Tubal Nuclear Norms. AAAI Conference on Artificial Intelligence (AAAI), 2020. [Pdf
link] (Oral)
[4] Wang, A., Song, X., Wu, X., Lai, Z., & Jin, Z. (2019). Latent Schatten TT norm for tensor completion. IEEE International Conference on Acoustics, Speech and Signal Processing (ICASSP), 2019. (Oral)
[3] Wang, A., Lai, Z., & Jin, Z. (2019). Noisy low-tubal-rank tensor completion. Neurocomputing, 330, 267-279.
[2] Wang, A., Wei, D., Wang, B., & Jin, Z. (2018). Noisy low-tubal-rank tensor completion through iterative singular tube thresholding. IEEE Access, 6, 35112-35128.
[Pdf
link]
[1] Liu, J., Tang, Z., Wang, A., & Shi, C. (2017). Negative obstacle detection in unstructured environment based on multiple LiDARs and compositional features. Jiqiren/Robot. (in Chinese) [Pdf
link]